OPEN ACCESS
Article Peer-Reviewed
Open
Peer Review
Peer Review
Optimization of Traffic Accident Quantity Estimation Method Synergy of Factors Affecting Traffic Accident Quantity with Raw Values
Stanislaw Staszic State University of Applied Sciences in Pila, ul. Podchorazych 10, 64-920 Pila, Poland
*
For correspondence.
Academic Editor:
Received: 19 September 2023 Accepted: 29 December 2023 Published: 13 February 2024
Abstract
As the number of vehicles on the road increases, traffic accidents are becoming more destructive, causing loss of life and work. This is due to rapid population growth and the development of motorization. The most important challenge in estimating and studying information about street twists of fate is the small amount of facts available for this analysis. Although car accidents kill and injure millions of people around the world each year, they are rare in time and space. The motive of this article is to advise an effective approach to estimating the number of accidents on Poland’s roads, based primarily on a combination of factors affecting such layered situations. The methodology presented in this paper for the use of multi-criteria optimization procedures using a multi-criteria optimization model (a set of forecasting methods, sub-criteria of the criterion function, and elements of the dominance relationship) allows us to conclude that the above methodology can be used to optimize methods for forecasting road accidents in Poland.
Figures in this Article
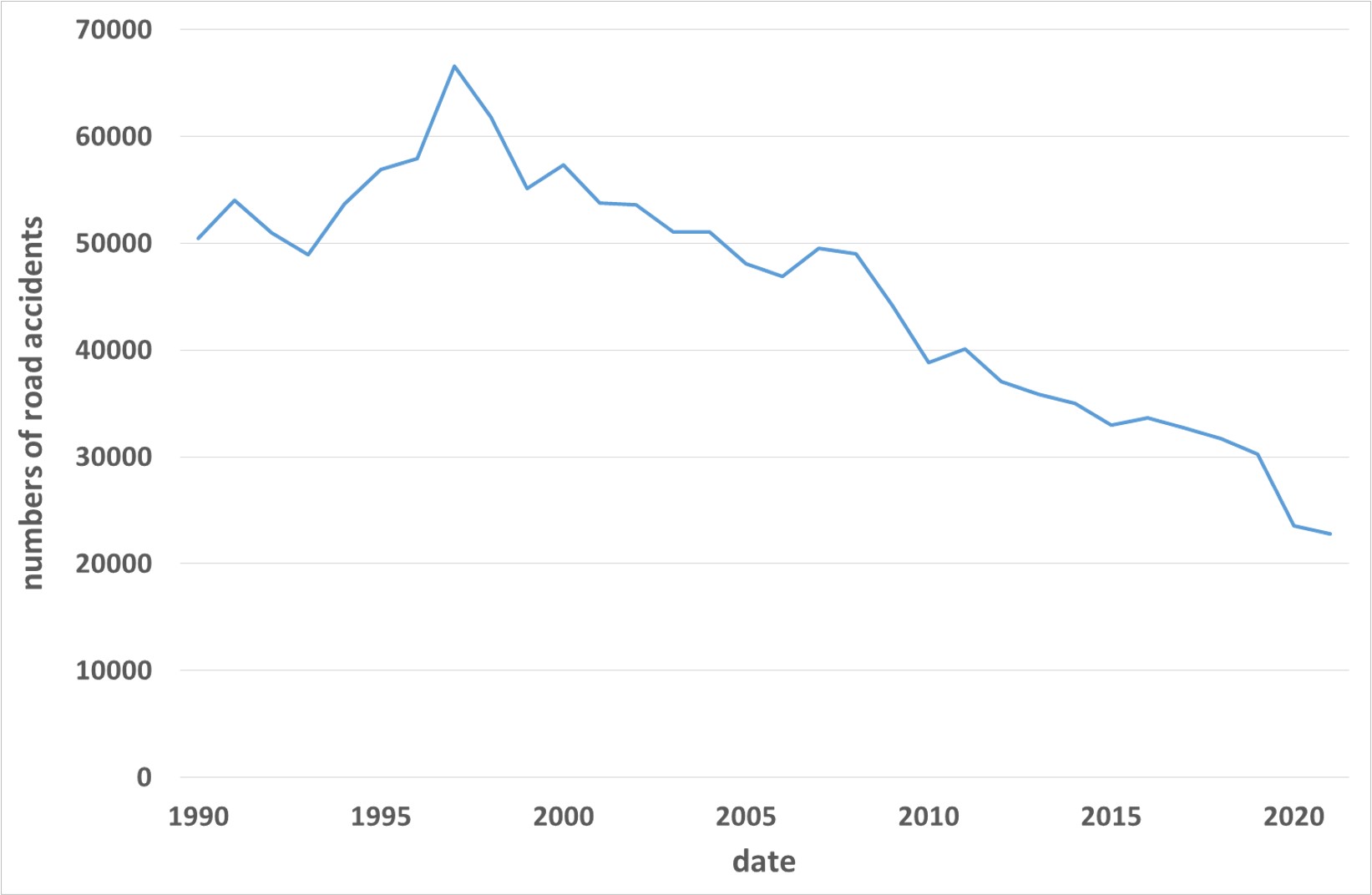
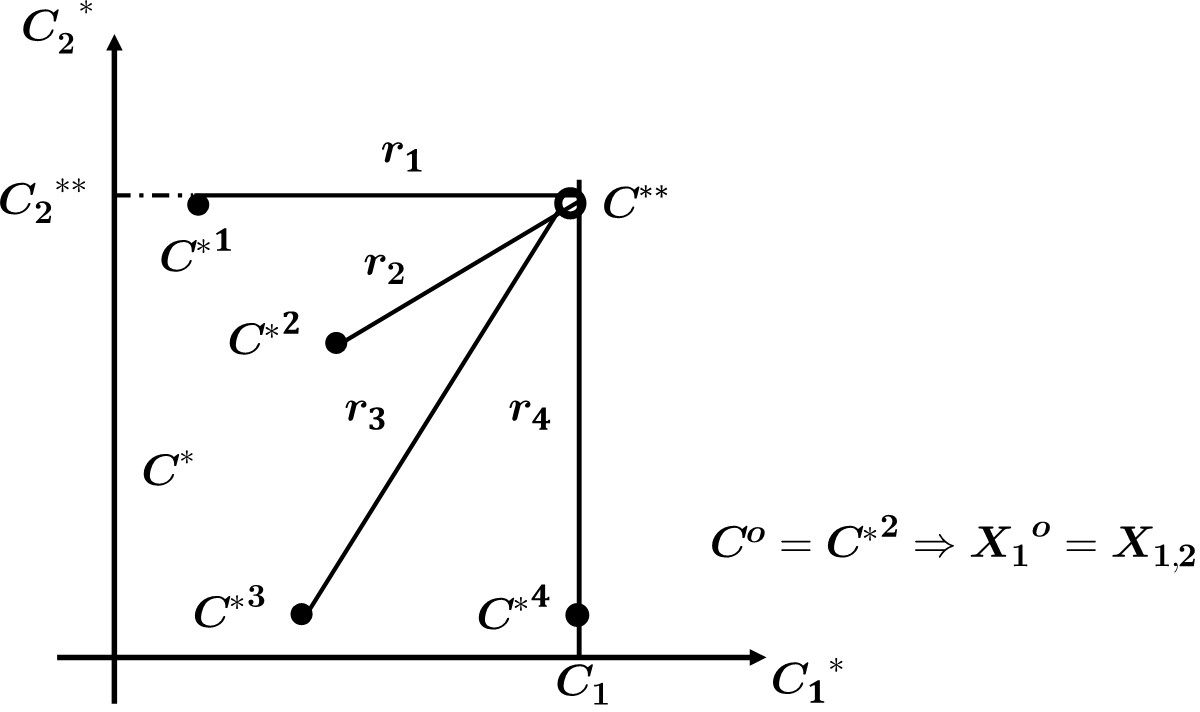
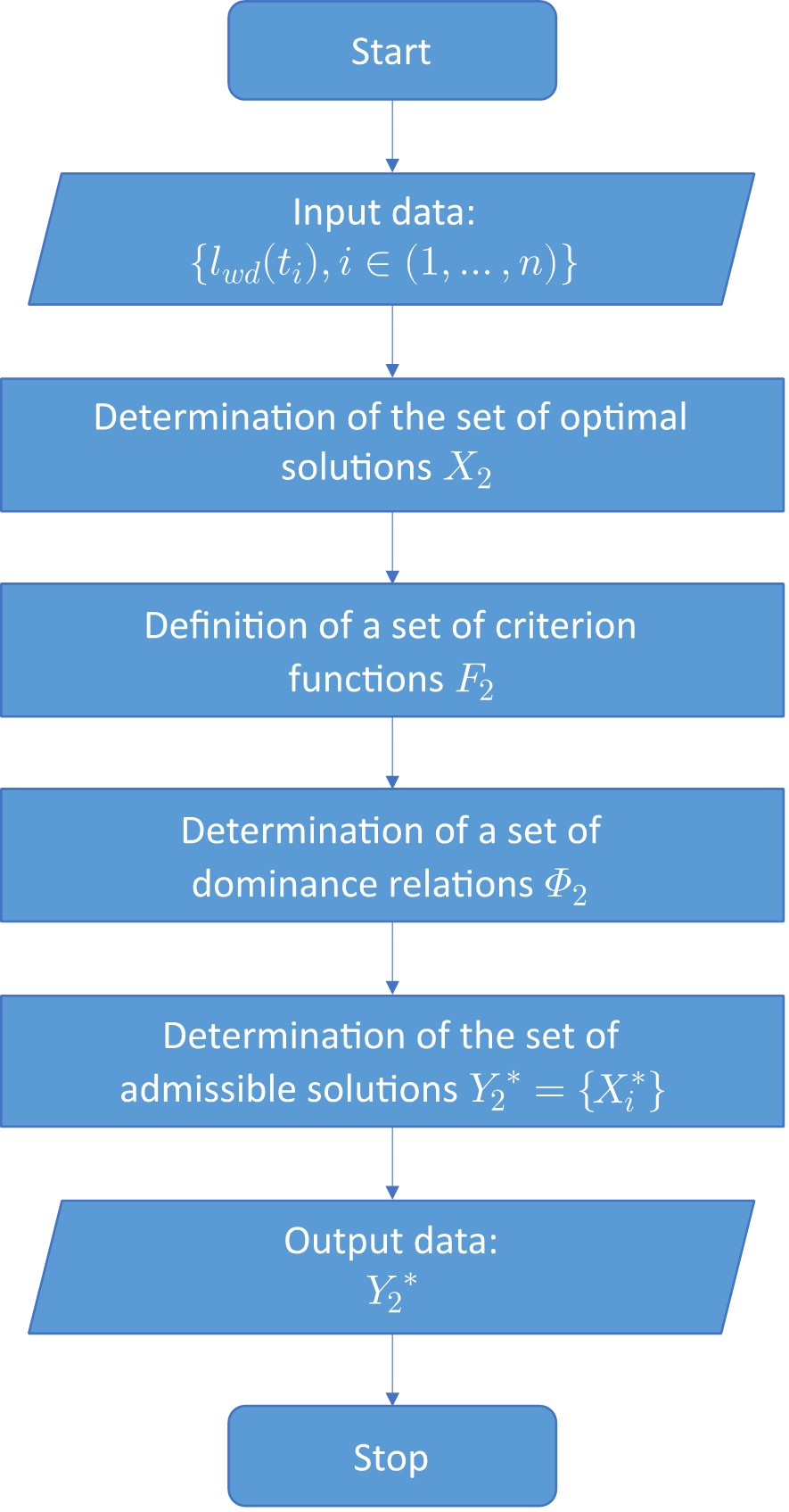
Keywords
traffic accidents; the synergy of factors affecting the variety of traffic accidents; forecasting methods; multi-criteria optimization
Copyright © 2024
Gorzelanczyk and Tylicki. This article is distributed under the terms of the Creative Commons Attribution License (CC BY 4.0), which permits unrestricted use and distribution provided that the original work is properly cited.
Funding
The article was financed by the university’s own funds.
Cite this Article
Gorzelanczyk, P., & Tylicki, H. (2024). Optimization of Traffic Accident Quantity Estimation Method Synergy of Factors Affecting Traffic Accident Quantity with Raw Values. Highlights of Vehicles, 2(1), 1–12. https://doi.org/10.54175/hveh2010001
References
1.
World Health Organization. (2020). Global status report on road safety 2020. https://www.who.int/violence_injury_prevention/road_safety_status/report/en (accessed 10 May 2022).
2.
European Union. (2022). Eurostat Statistics. https://ec.europa.eu/eurostat (accessed 10 May 2022).
3.
Statystyka. (2022). Police Statistics. https://statystyka.policja.pl (accessed 10 May 2022).
4.
Tambouratzis, T., Souliou, D., Chalikias, M., & Gregoriades, A. (2014). Maximising accuracy and efficiency of traffic accident prediction combining information mining with computational intelligence approaches and decision trees. Journal of Artificial Intelligence and Soft Computing Research, 4(1), 31–42. https://doi.org/10.2478/jaiscr-2014-0023
5.
Zhu, L., Lu, L., Zhang, W., Zhao, Y., & Song, M. (2019). Analysis of accident severity for curved roadways based on Bayesian networks. Sustainability, 11(8), 2223. https://doi.org/10.3390/su11082223
6.
Arteaga, C., Paz, A., & Park, J. (2020). Injury severity on traffic crashes: A text mining with an interpretable machine-learning approach. Safety Science, 132, 104988. https://doi.org/10.1016/j.ssci.2020.104988
7.
Yang, Z., Zhang, W., & Feng, J. (2022). Predicting multiple types of traffic accident severity with explanations: A multi-task deep learning framework. Safety Science, 146, 105522. https://doi.org/10.1016/j.ssci.2021.105522
8.
Gorzelanczyk, P., Pyszewska, D., Kalina, T., & Jurkovic, M. (2020). Analysis of road traffic safety in the Pila poviat. Scientific Journal of Silesian University of Technology. Series Transport, 107, 33–52. https://doi.org/10.20858/sjsutst.2020.107.3
9.
Chen, C. (2017). Analysis and forecast of traffic accident big data. ITM Web of Conferences, 12, 04029. https://doi.org/10.1051/itmconf/20171204029
10.
Khaliq, K. A., Chughtai, O., Shahwani, A., Qayyum, A., & Pannek, J. (2019). Road accidents detection, data collection and data analysis using V2X communication and edge/cloud computing. Electronics, 8(8), 896; https://doi.org/10.3390/electronics8080896
11.
Rajput, H., Som, T., & Kar, S. (2015). An automated vehicle license plate recognition system. Computer, 48(8), 56–61. https://doi.org/10.1109/MC.2015.244
12.
Zheng, Z., Wang, C., Wang, P., Xiong, Y., Zhang, F., & Lv, Y. (2018). Framework for fusing traffic information from social and physical transportation data. PLOS ONE, 13(8), e0201531. https://doi.org/10.1371/journal.pone.0201531
13.
Abdullah, E., & Emam, A. (7–9 December 2015). Traffic accidents analyzer using big data. 2015 International Conference on Computational Science and Computational Intelligence (CSCI), Las Vegas, NV, USA. https://doi.org/10.1109/CSCI.2015.187
14.
Vilaça, M., Silva, N., & Coelho, M. C. (2017). Statistical analysis of the occurrence and severity of crashes involving vulnerable road users. Transportation Research Procedia, 27, 1113–1120. https://doi.org/10.1016/j.trpro.2017.12.113
15.
Bąk, I., Cheba, K., & Szczecińska, B. (2019). The statistical analysis of road traffic in cities of Poland. Transportation Research Procedia, 39, 14–23. https://doi.org/10.1016/j.trpro.2019.06.003
16.
Chand, A., Jayesh, S., & Bhasi, A. B. (2021). Road traffic accidents: An overview of data sources, analysis techniques and contributing factors. Materials Today: Proceedings, 47(15), 5135–5141. https://doi.org/10.1016/j.matpr.2021.05.415
17.
Wójcik, A. (2014). Autoregressive vector models as a response to the critique of multi-equation structural econometric models. University of Economics in Katowice.
18.
Biswas, A. A., Mia, M. J., & Majumder, A. (6–8 July 2019). Forecasting the Number of Road Accidents and Casualties using Random Forest Regression in the Context of Bangladesh. 2019 10th International Conference on Computing, Communication and Networking Technologies (ICCCNT), Kanpur, India. https://doi.org/10.1109/ICCCNT45670.2019.8944500
19.
Wikipedia. (2022). Random forest. https://en.wikipedia.org/wiki/Random_forest (accessed 10 May 2022).
20.
Fijorek, K., Mróz, K., Niedziela, K., & Fijorek, D. (2010). Forecasting electricity prices on the day-ahead market using data mining methods (in Polish). Energy Market.
21.
Chudy-Laskowska, K., & Pisula, T. (2015). Forecasting the number of road accidents in Podkarpaciu (in Polish). Logistyka, 2, 2782–2796.
22.
Procházka, J., Flimmel, S., Čamaj, M., & Bašta, M. (30 August–3 September 2017). Modelling the Number of Road Accidents. 20-th AMSE. Applications of Mathematics and Statistics in Economics. International Scientific Conference: Szklarska Poręba, Poland. https://doi.org/10.15611/amse.2017.20.29
23.
Sunny, C. M., Nithya, S., https://ieeexplore.ieee.org/author/37086511696, K. S., Vinodini, V., Lakshmi, A., Anjana, S., et al. (7–9 August 2018). Forecasting of Road Accident in Kerala: A Case Study. 2018 International Conference on Data Science and Engineering (ICDSE), Kochi, India. https://doi.org/10.1109/ICDSE.2018.8527825
24.
Dutta, B., Barman, M. P., & Patowary, A. N. (2020). Application of Arima model for forecasting road accident deaths in India. International Journal of Agricultural and Statistical Sciences, 16(2), 607–615.
25.
Karlaftis, M. G., & Vlahogianni, E. I. (2009). Memory properties and fractional integration in transportation time-series. Transportation Research Part C: Emerging Technologies, 17(4), 444–453. https://doi.org/10.1016/j.trc.2009.03.001
26.
Łobejko, S. (2015). Time series analysis and forecasting with SAS. Main business school in Warsaw.
27.
Dudek, G. (2013). Forecasting Time Series with Multiple Seasonal Cycles Using Neural Networks with Local Learning. In L. Rutkowski, M. Korytkowski, R. Scherer, R. Tadeusiewicz, L. A. Zadeh, & J. M. Zurada (Eds.), Artificial Intelligence and Soft Computing. ICAISC 2013. Lecture Notes in Computer Science (Vol. 7894). Springer. https://doi.org/10.1007/978-3-642-38658-9_5
28.
StatSoft. (2022). Data Mining Techniques (in Polish). https://www.statsoft.pl/textbook/stathome_stat.html?https%3A%2F%2Fwww.statsoft.pl%2Ftextbook%2Fstdatmin.html (accessed 10 May 2022).
29.
Kumar, P. S., Viswanadham, V., & Bharathi, B. (2019). Analysis of road accident. IOP Conference Series Materials Science and Engineering, 590, 012029. https://doi.org/10.1088/1757-899X/590/1/012029
30.
DataFlair. (2022). Top Advantages and Disadvantages of Hadoop 3. https://data-flair.training/blogs/advantages-and-disadvantages-of-hadoop (accessed 10 May 2022).
31.
Perczak, G., & Fiszeder, P. (2014). GARCH model - using additional information on minimum and maximum prices (in Polish). Bank and Credit.
32.
McIlroy, R. C., Plant, K. A., Hoque, M. S., Wu, J., Kokwaro, G. O., Nam, V. H., et al. (2019). Who is responsible for global road safety? A cross-cultural comparison of actor maps. Accident Analysis & Prevention, 122, 8–18. https://doi.org/10.1016/j.aap.2018.09.011
33.
Muck, J. (2022). Econometrics. Modeling of time series. Stationary. Unit root tests. ARDL models. Co-integration (in Polish). http://web.sgh.waw.pl/~jmuck/Ekonometria/EkonometriaPrezentacja5.pdf (accessed 10 May 2022).
34.
Patil, D., Franklin, R., Deshmukh, S., Pillai, S., & Nashipudimath, M. (2020) Analysis of road accidents using data mining techniques. International Research Journal of Engineering and Technology, 7(5), 6859–6862.
35.
Li, L., Shrestha, S., & Hu, G. (7–9 June 2017). Analysis of road traffic fatal accidents using data mining techniques. 2017 IEEE 15th International Conference on Software Engineering Research, Management and Applications (SERA), London, UK. https://doi.org/10.1109/SERA.2017.7965753
36.
Marcinkowska, J. (2015). Statistical methods and data mining in assessing the occurrence of syncope in the group of narrow-QRS tachycardia [PhD Thesis, Medical University of Karol Marcinkowski in Poznań] (in Polish). Digital Library of Wielkopolska. http://www.wbc.poznan.pl/Content/373785/index.pdf (accessed 10 May 2022).
37.
Sebego, M., Naumann, R. B., Rudd, R. A., Voetsch, K., Dellinger, A. M., & Ndlovu, C. (2014). The impact of alcohol and road traffic policies on crash rates in Botswana, 2004–2011: a time-series analysis. Accident Analysis & Prevention, 70, 33–39. https://doi.org/10.1016/j.aap.2014.02.017
38.
Bloomfield, P. (1973). An exponential model in the spectrum of a scalar time series. Biometrika, 60(2), 217–226. https://doi.org/10.2307/2334533
39.
Ameljańczyk, A. (1986). Multi-criteria optimization. Military University of Technology.
40.
Tylicki, H., & Gorzelańczyk, P. (2013). The use of condition forecasting methods in the logistics of means of transport (in Polish). Loistyka, 1, 2–6.
41.
Tylicki, H., & Gorzelańczyk, P. (2014). Automation of the process of monitoring the condition of means of transport (in Polish). Loistyka, 6, 10766–10775.
42.
Gorzelanczyk, P., Tylicki, H., Kalina, T., & Jurkovič, M. (2021). Optimizing the Choice of Means of Transport using Operational Research. Communications - Scientific letters of the University of Zilina, 23(3), A193–A207. https://doi.org/10.26552/com.C.2021.3.A193-A207
43.
Tylicki, H. (22–23 March 2009). Optimization of the anthropotechnical system. Materials of the XXXVII Winter School of Reliability, Szczyrk, Poland.
44.
Bhandari, B., Lee, K.-T., Lee, G.-Y., Cho, Y.-M., & Ahn, S.-H. (2015). Optimization of hybrid renewable energy power systems: A review. International Journal of Precision Engineering and Manufacturing-Green Technology, 2, 99–112. https://doi.org/10.1007/s40684-015-0013-z
45.
Tylicki, H. (2022). A model of optimizing the company’s energy management. In Materials GWDA Piła. GWDA Piła.
Metrics
Loading...
Journal Menu
Journal Contact
Highlights of Vehicles
Editorial Office
Highlights of Science
Avenida Madrid, 189-195, 3-3
08014 Barcelona, Spain
08014 Barcelona, Spain
Zejian Zhang
Managing Editor