OPEN ACCESS
Article Peer-Reviewed
Open
Peer Review
Peer Review
Measuring Cyclist’s Inputs, the Kinematic and Dynamic Properties of a City Bicycle, and Estimating the Road Profile via Sensor Fusion
1
Laboratoire Perceptions, Interactions, Comportements Simulations des usagers de la route et de la rue (PICS-L), Components and Systems Department (COSYS), Gustave Eiffel University, Champs sur Marne 77420, France
2
Division of Bioeconomy and Health, Department of Material and Surface Design, RISE Research Institutes of Sweden, Stockholm SE-114 28, Sweden
*
For correspondence.
Academic Editor:
Received: 16 November 2022 Accepted: 1 February 2023 Published: 5 February 2023
Abstract
In this paper, we present the instrumentation of a city bicycle with different sensors and devices in order to measure cyclists’ inputs (i.e., pedaling and steering) and the dynamical and kinematic properties of the bicycle. The instrumentation includes two tri-axial accelerometers, an Inertial Measurement Unit (IMU), GPS, a potentiometer, a laser scanner, a pedaling power meter, and speed and cadence sensors, in addition to a mobile eye tracker worn by the cyclists. After the instrumentation and adjustment of the sensors, a study was conducted in the city of Stockholm using the instrumented bicycle with the aim to evaluate cycling safety and comfort on snowy surface conditions. The outputs of this experiment will be employed further to study the interaction of cyclists with road infrastructure and other road users and their impact on cyclists’ behavior and cycling safety.
Figures in this Article
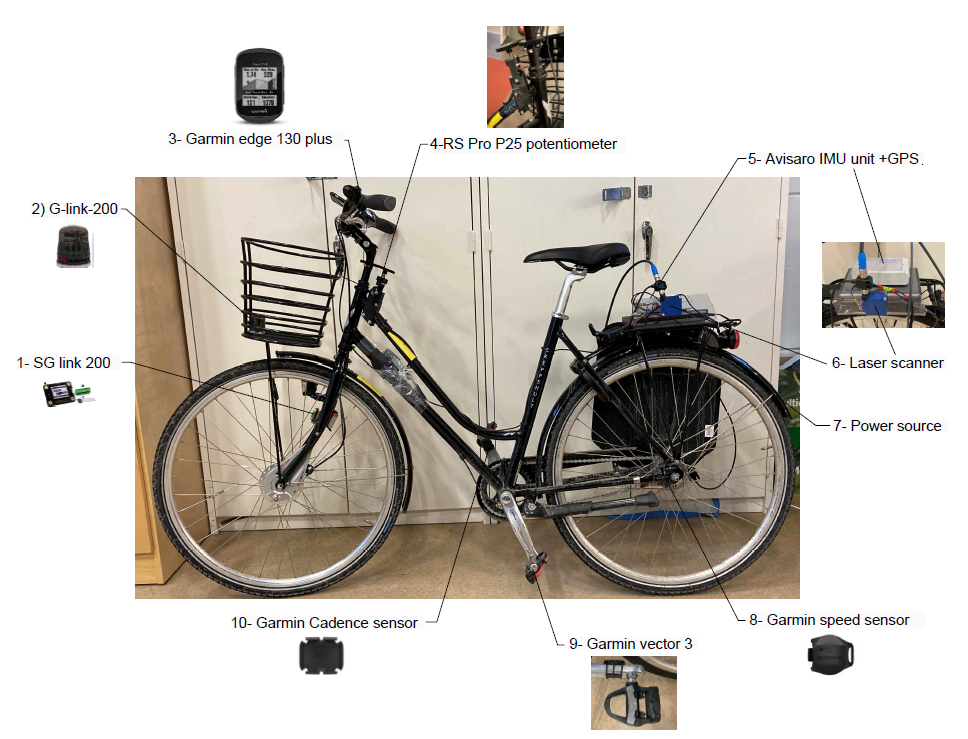
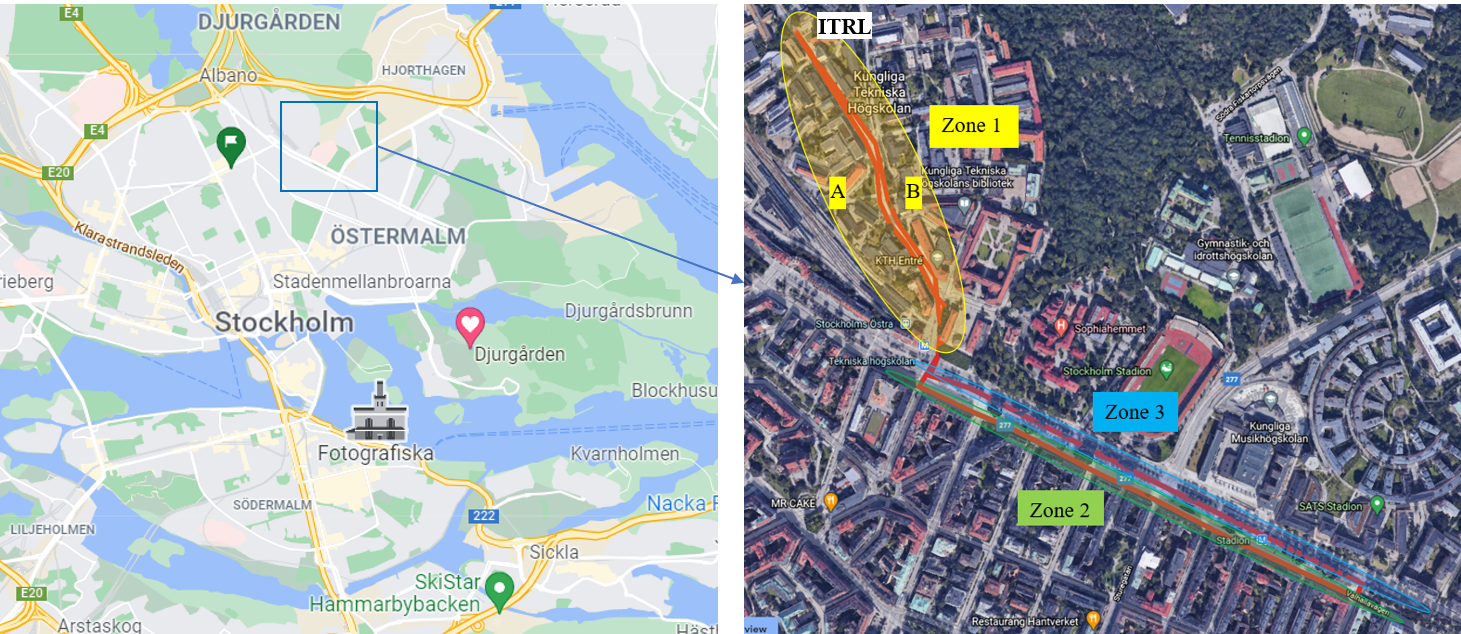
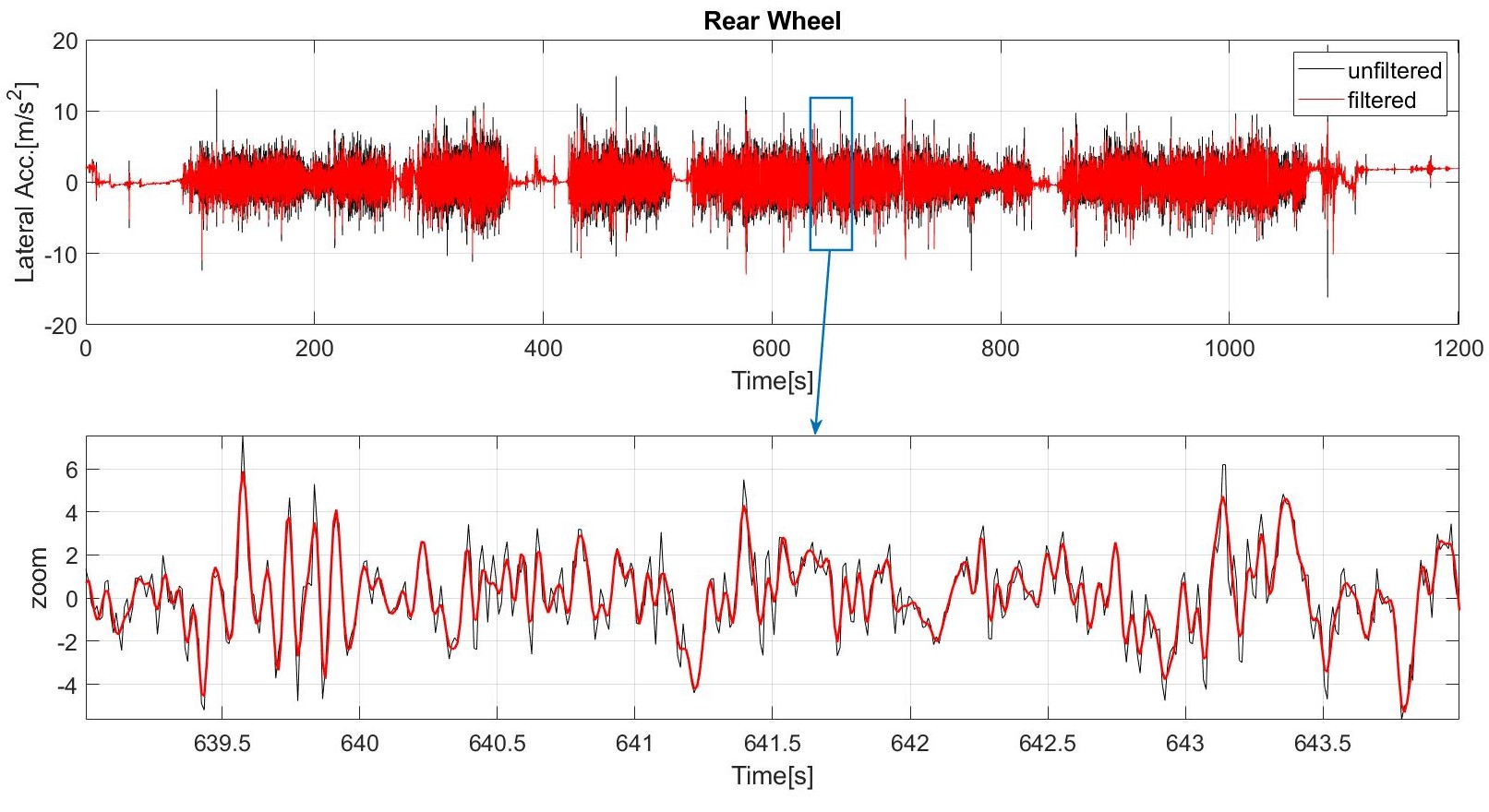
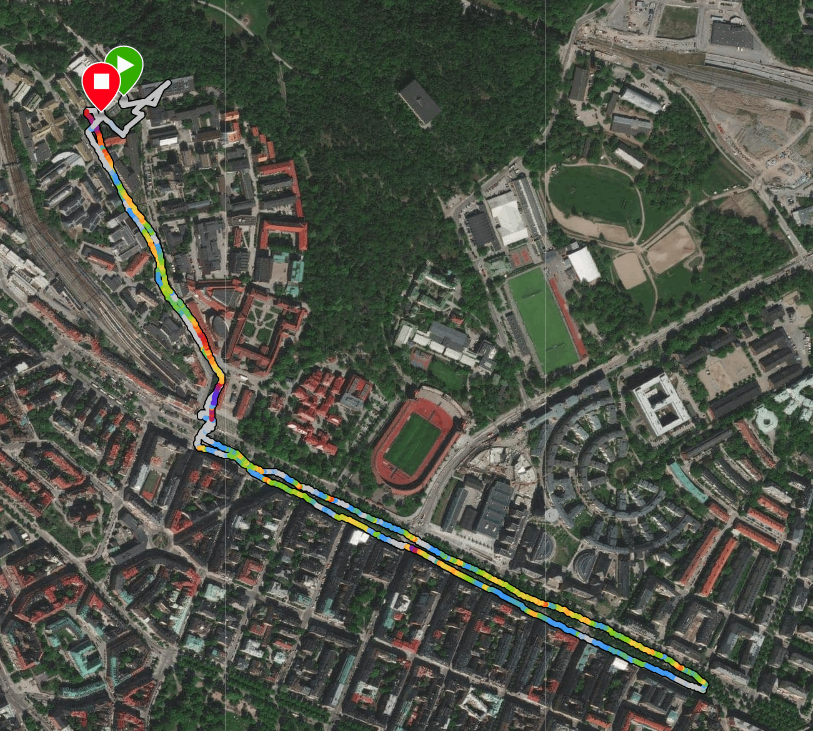
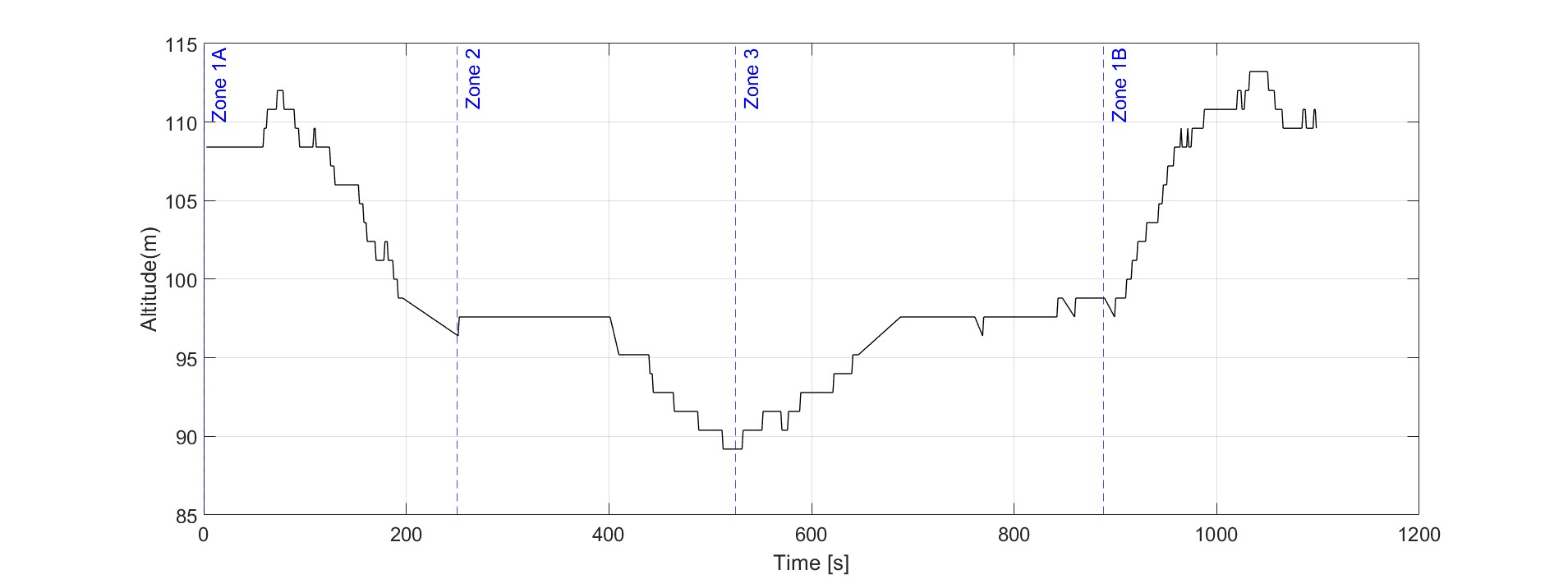
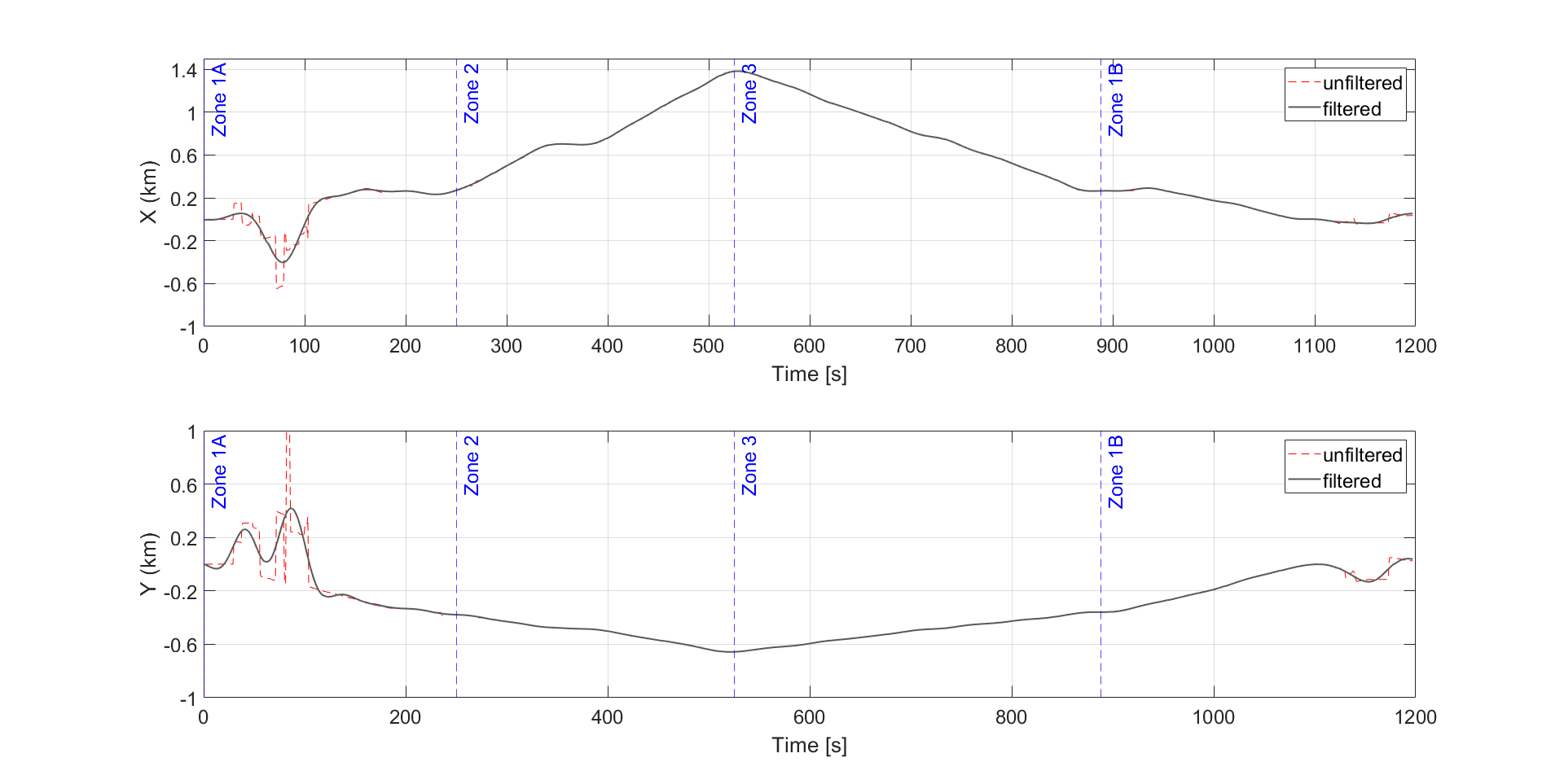
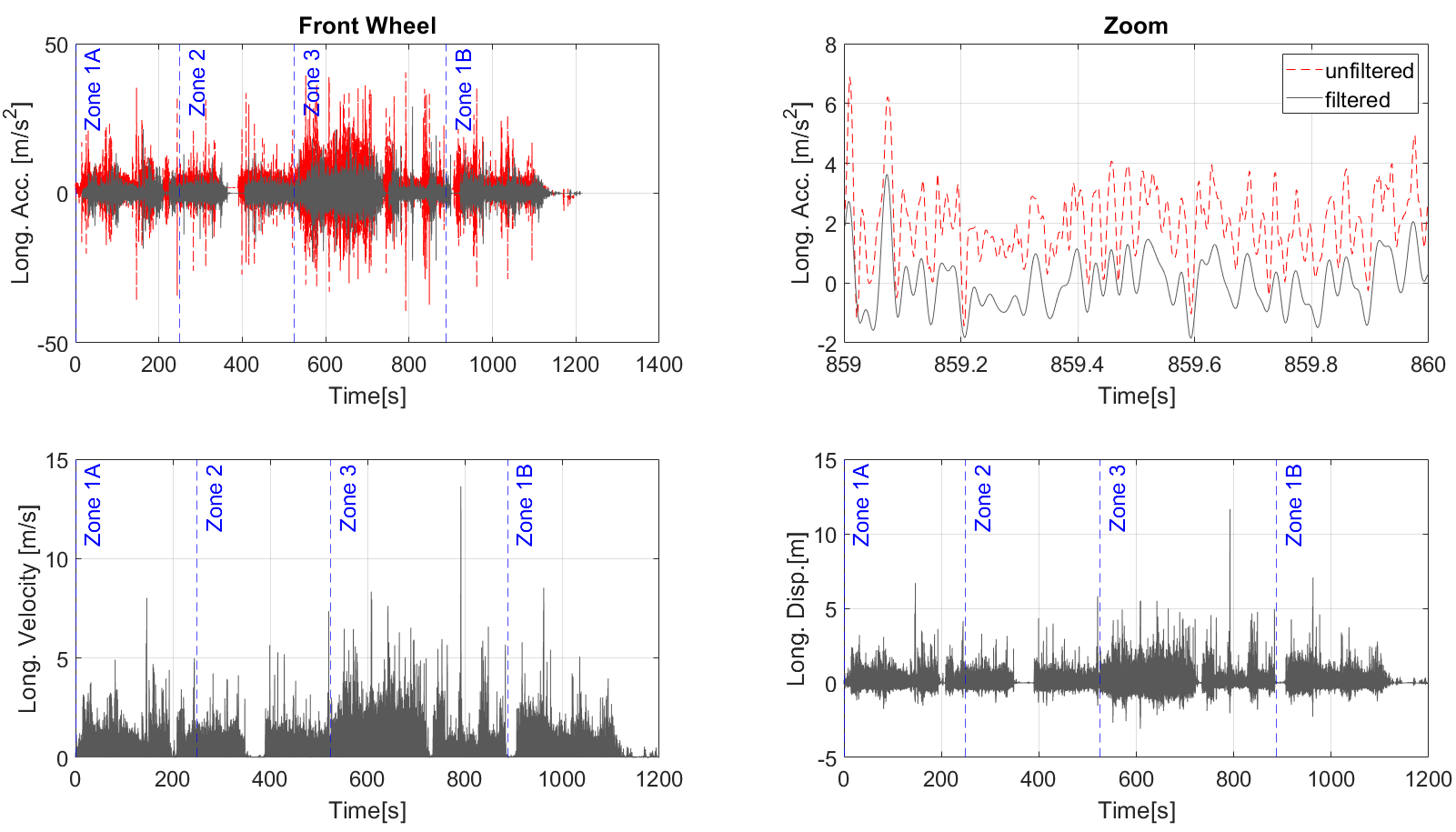
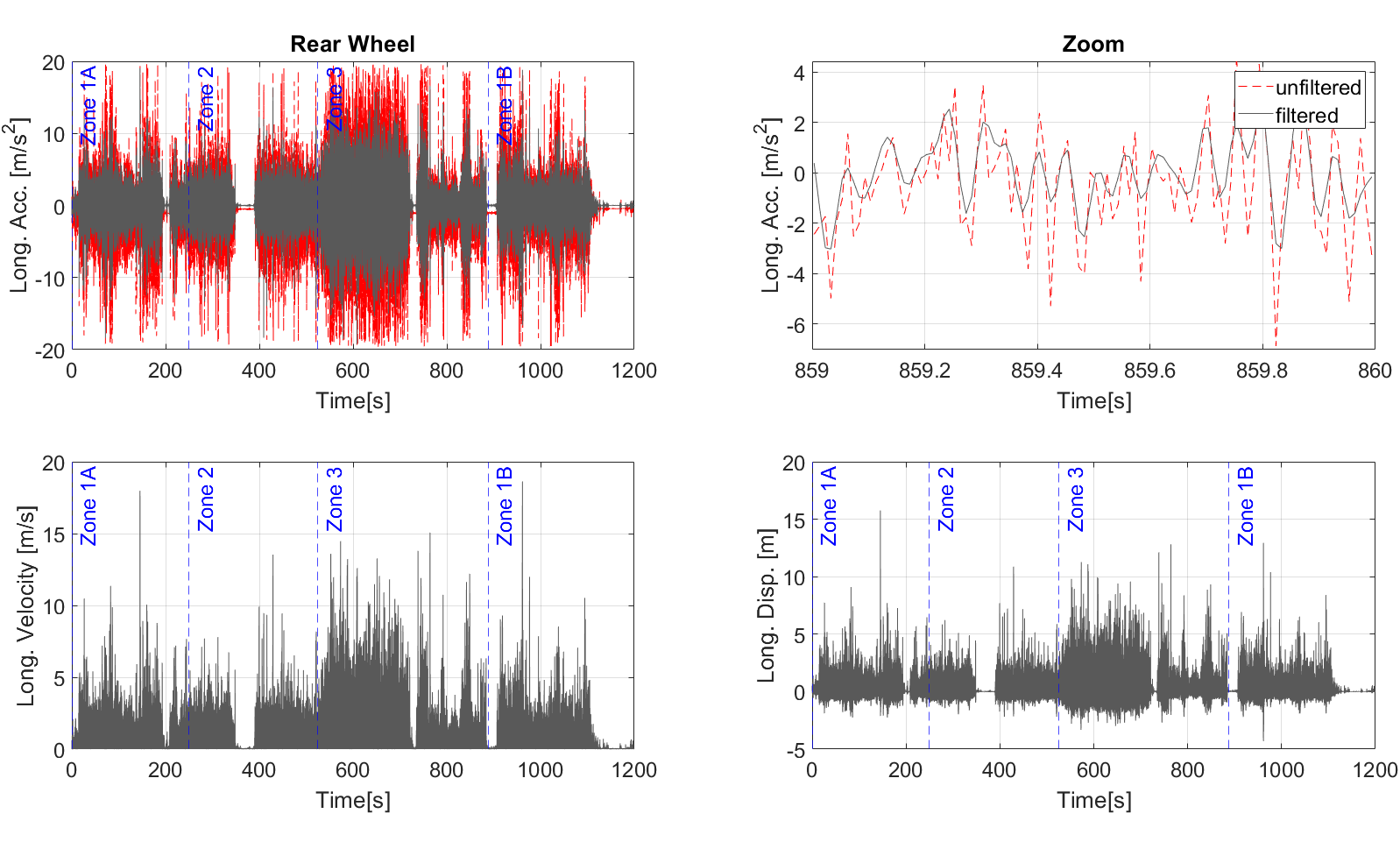
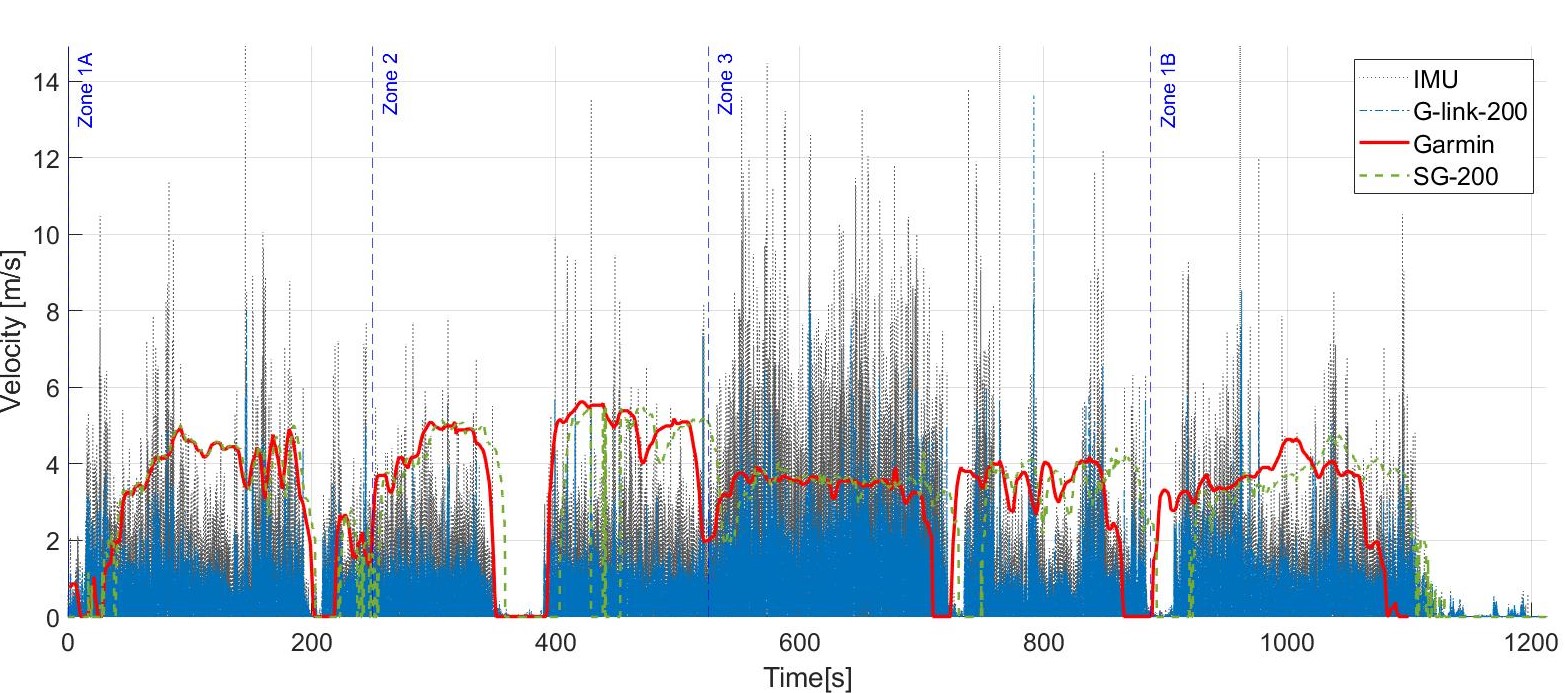
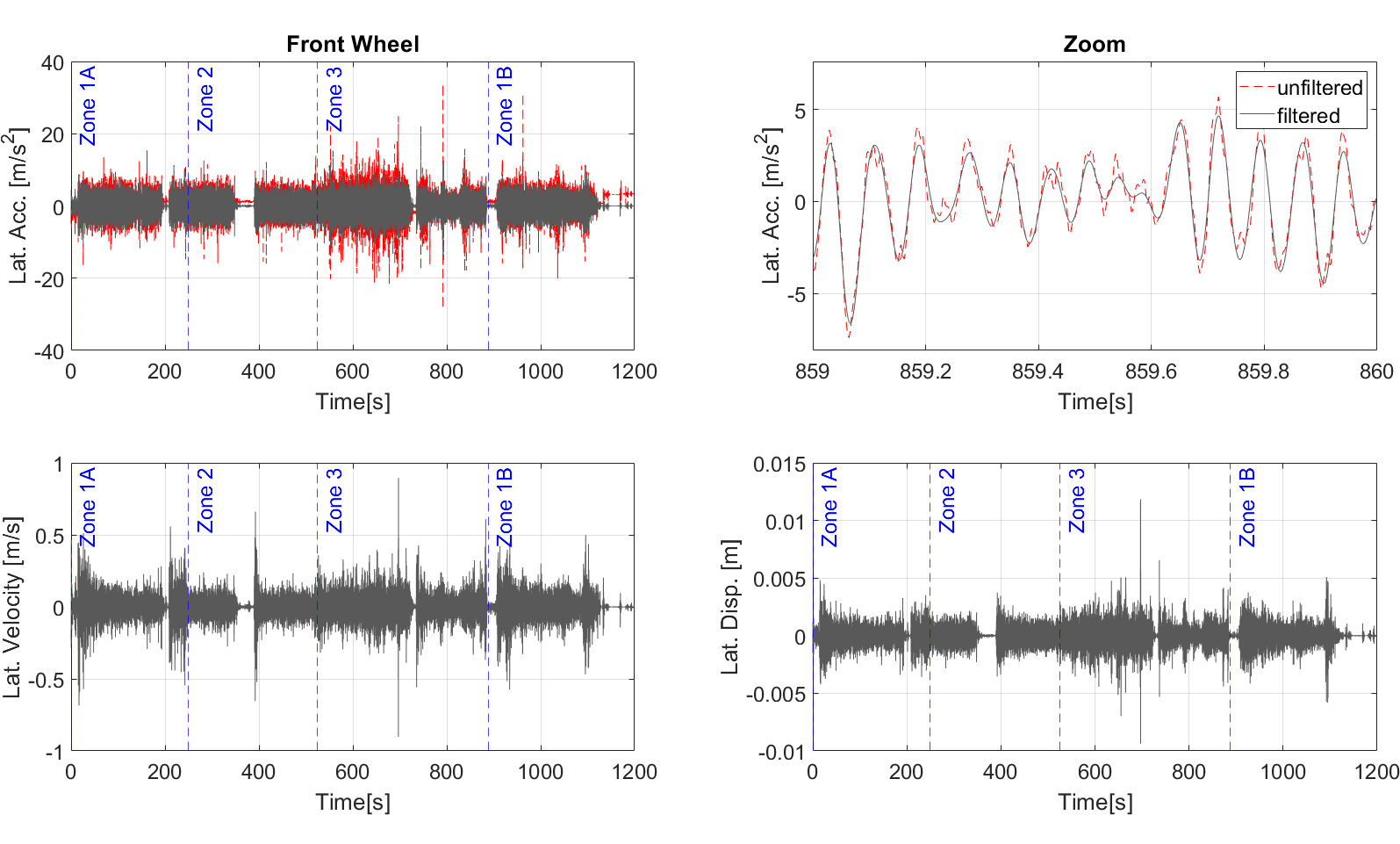
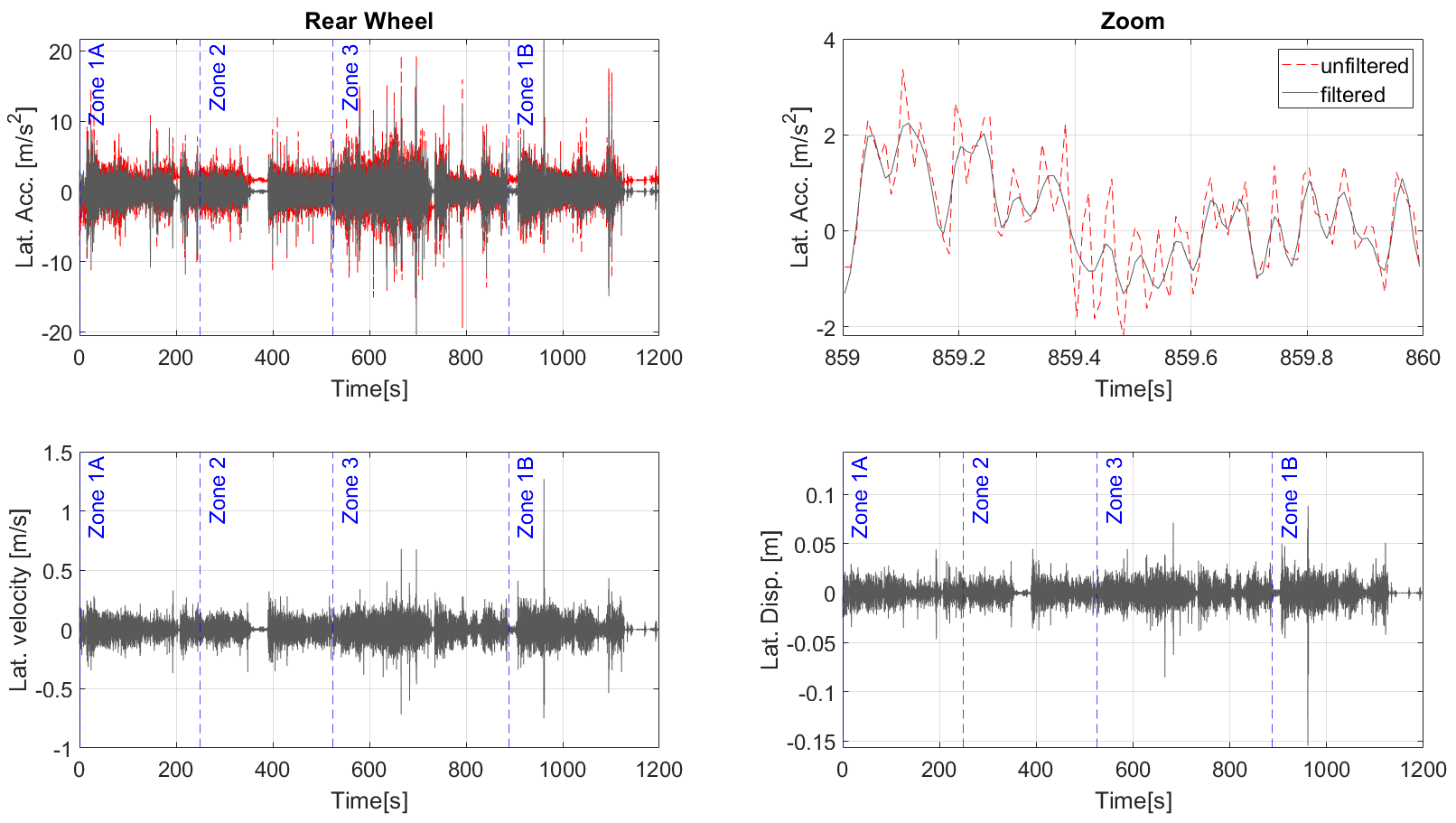
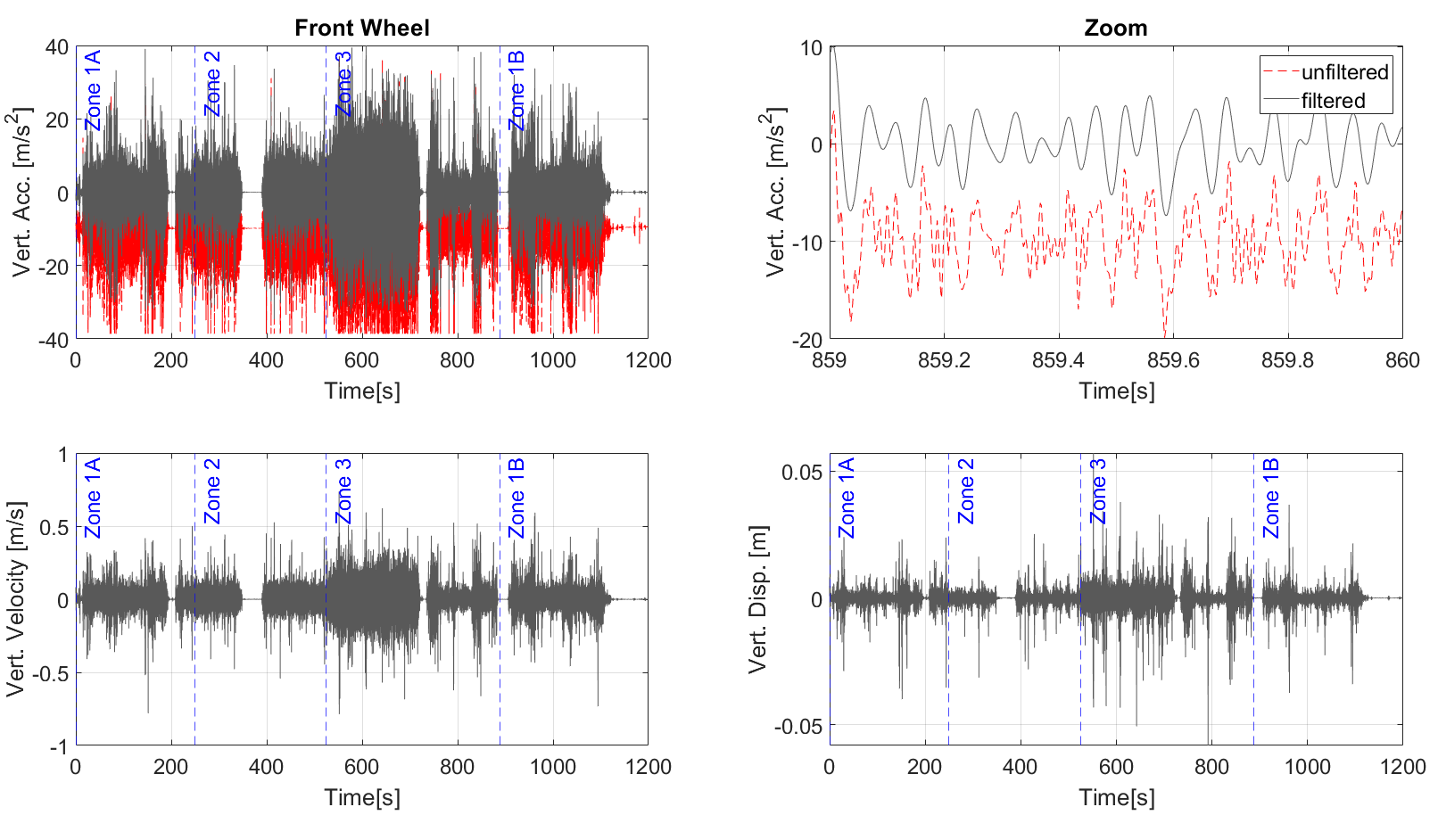
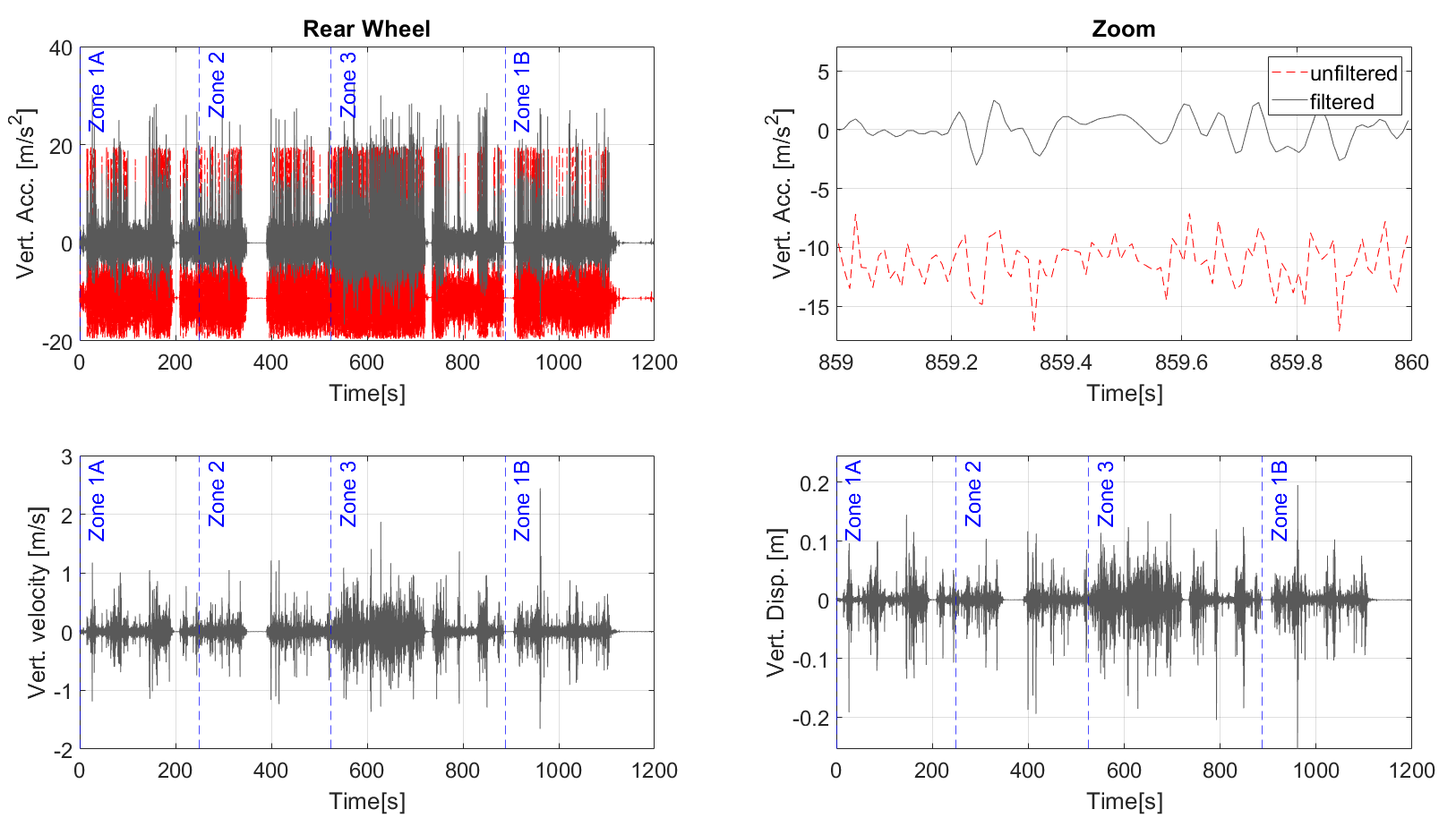
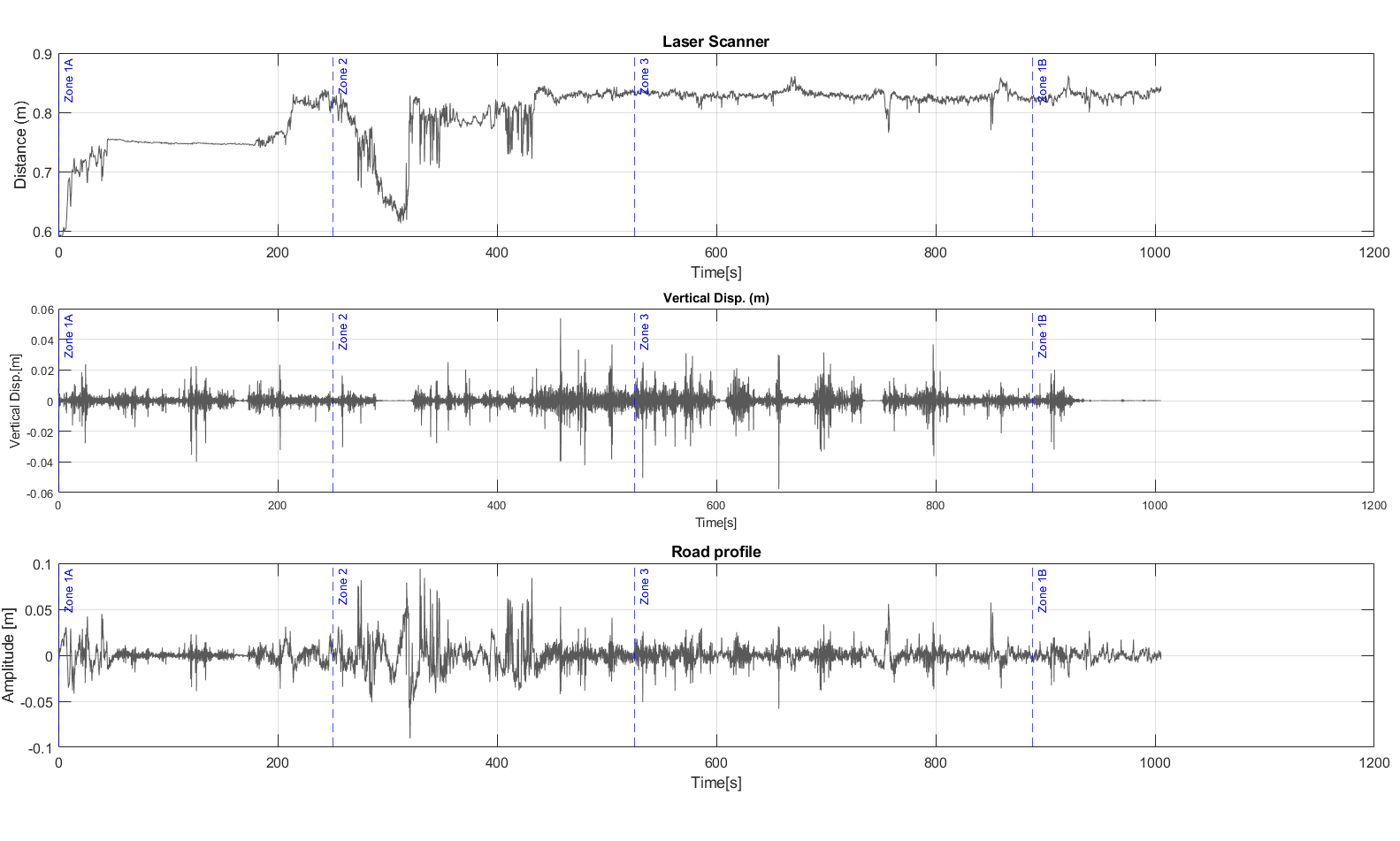
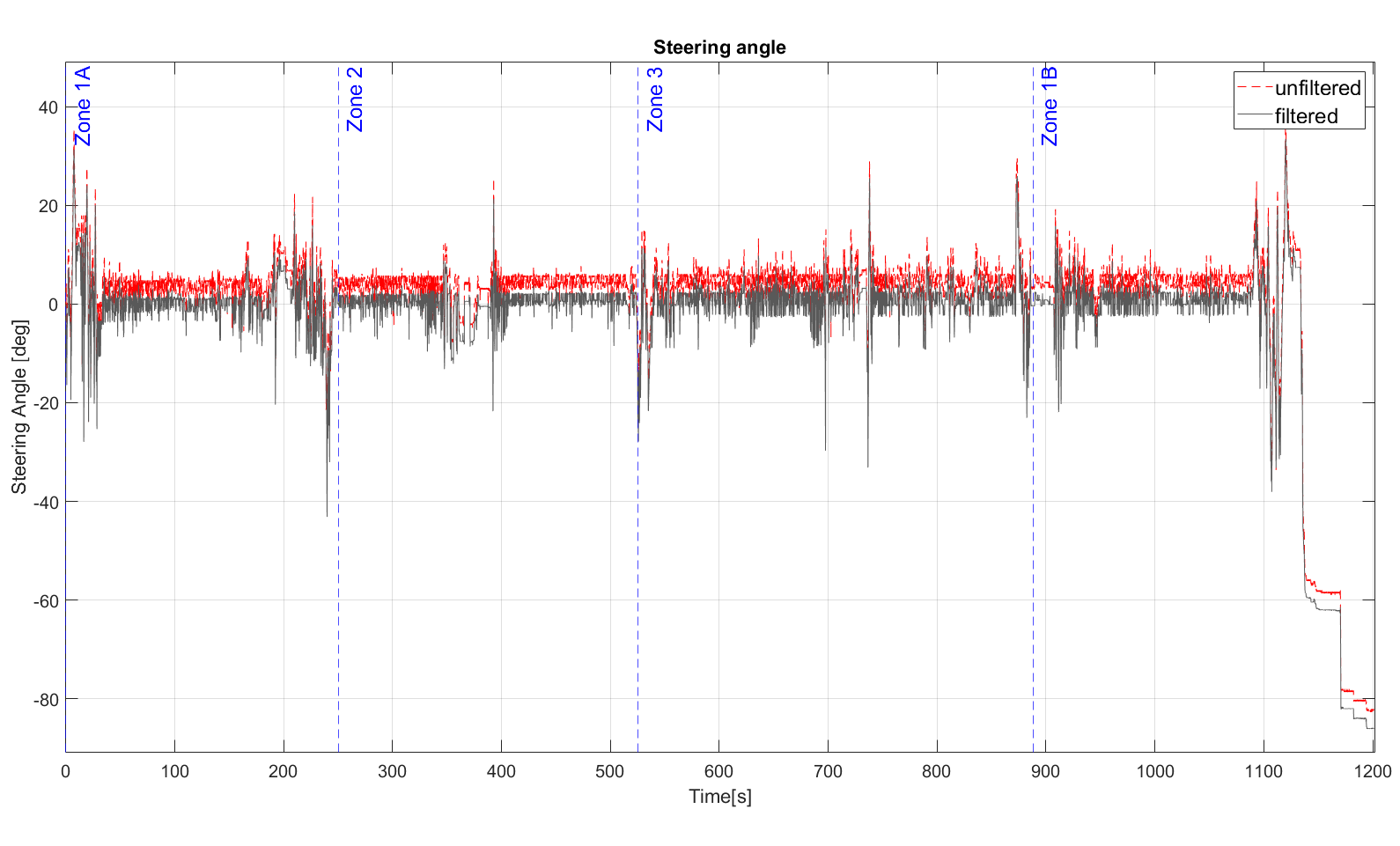
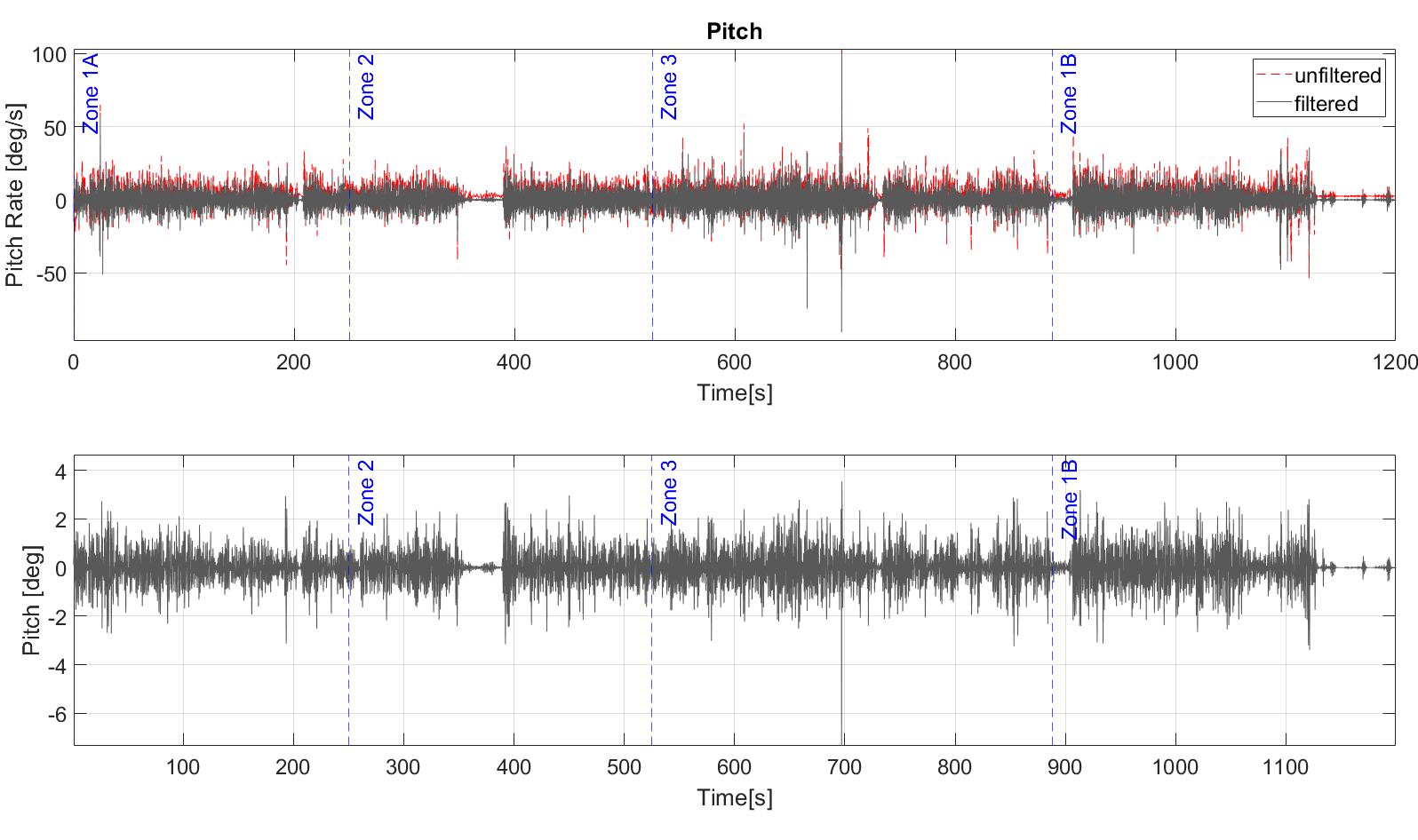
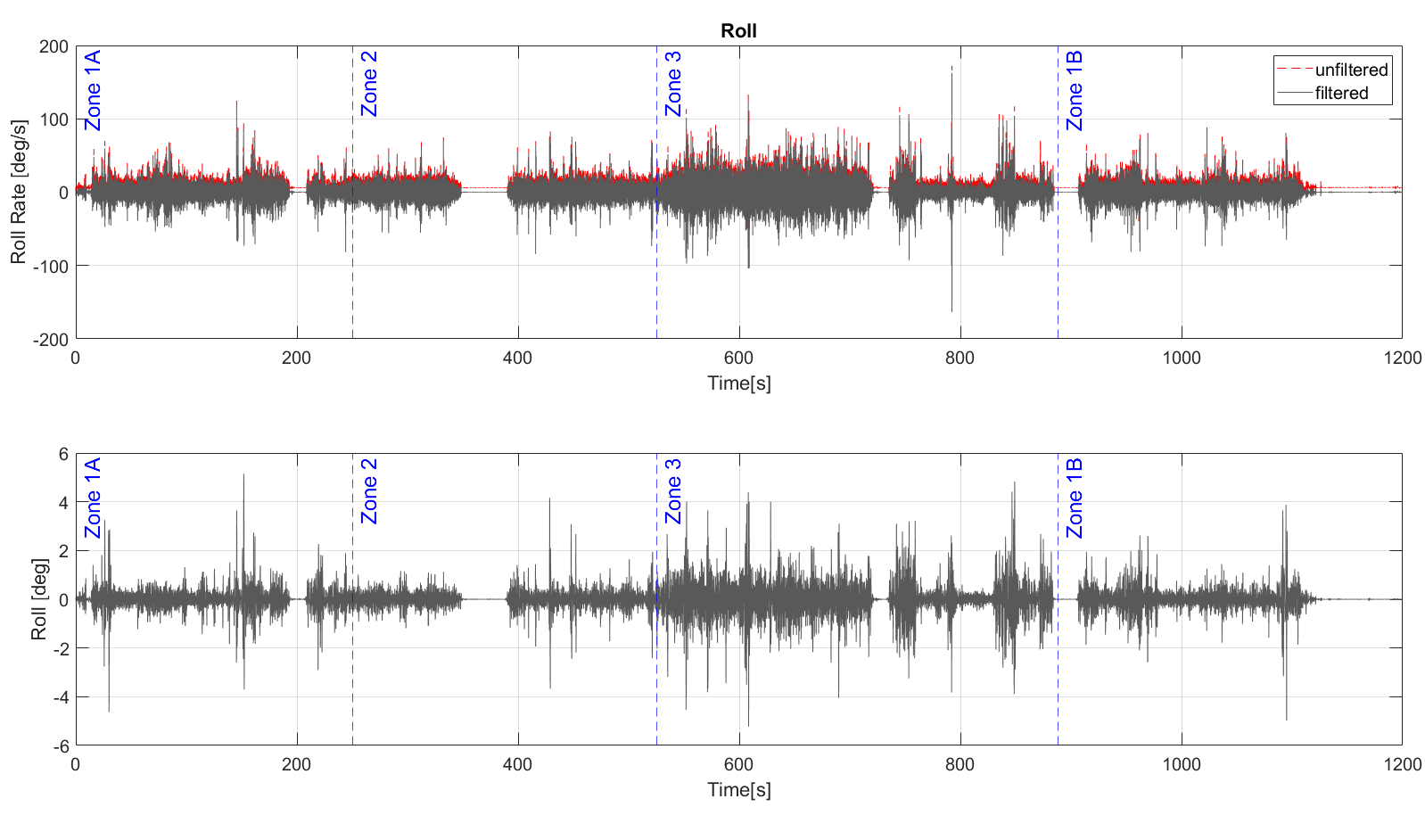
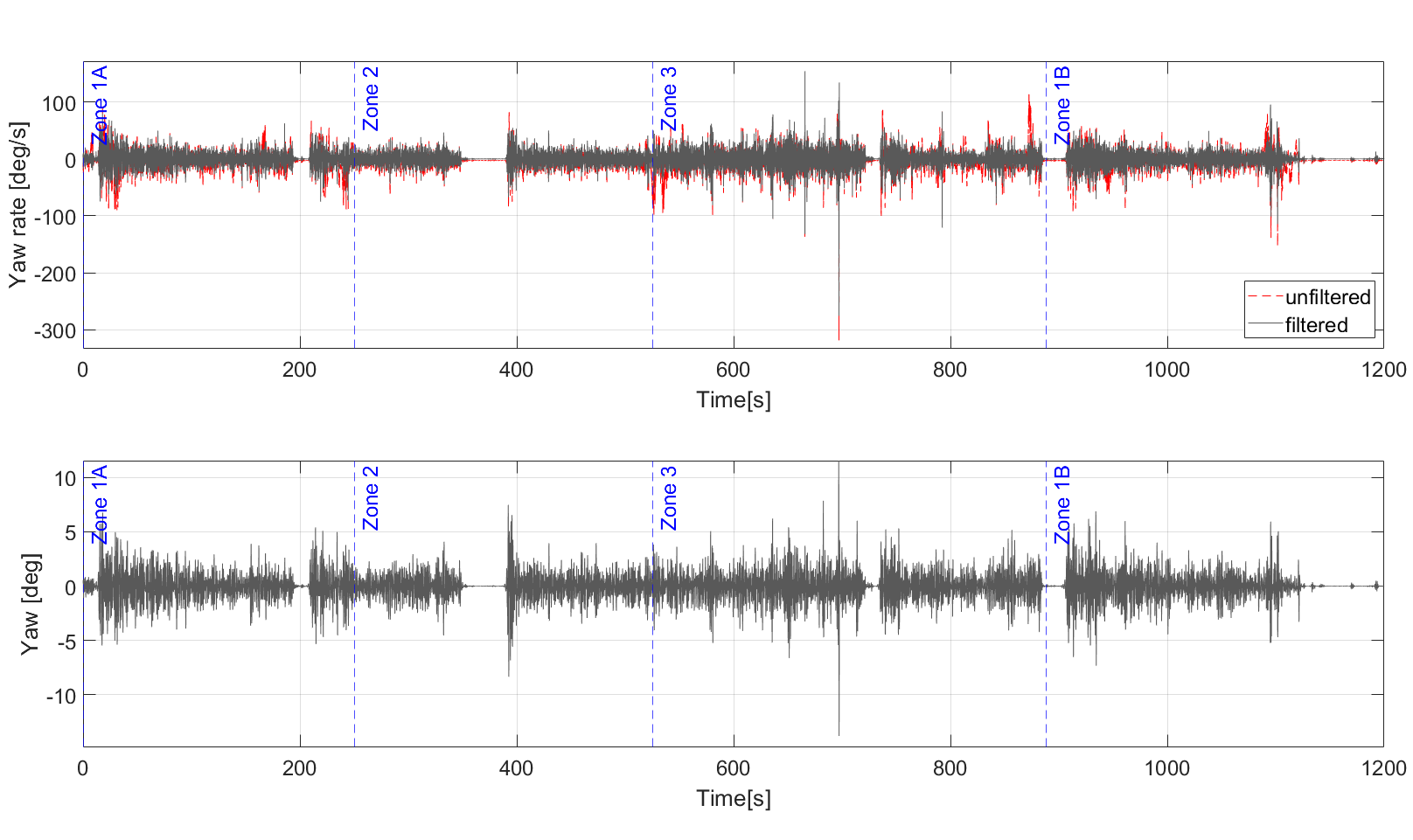
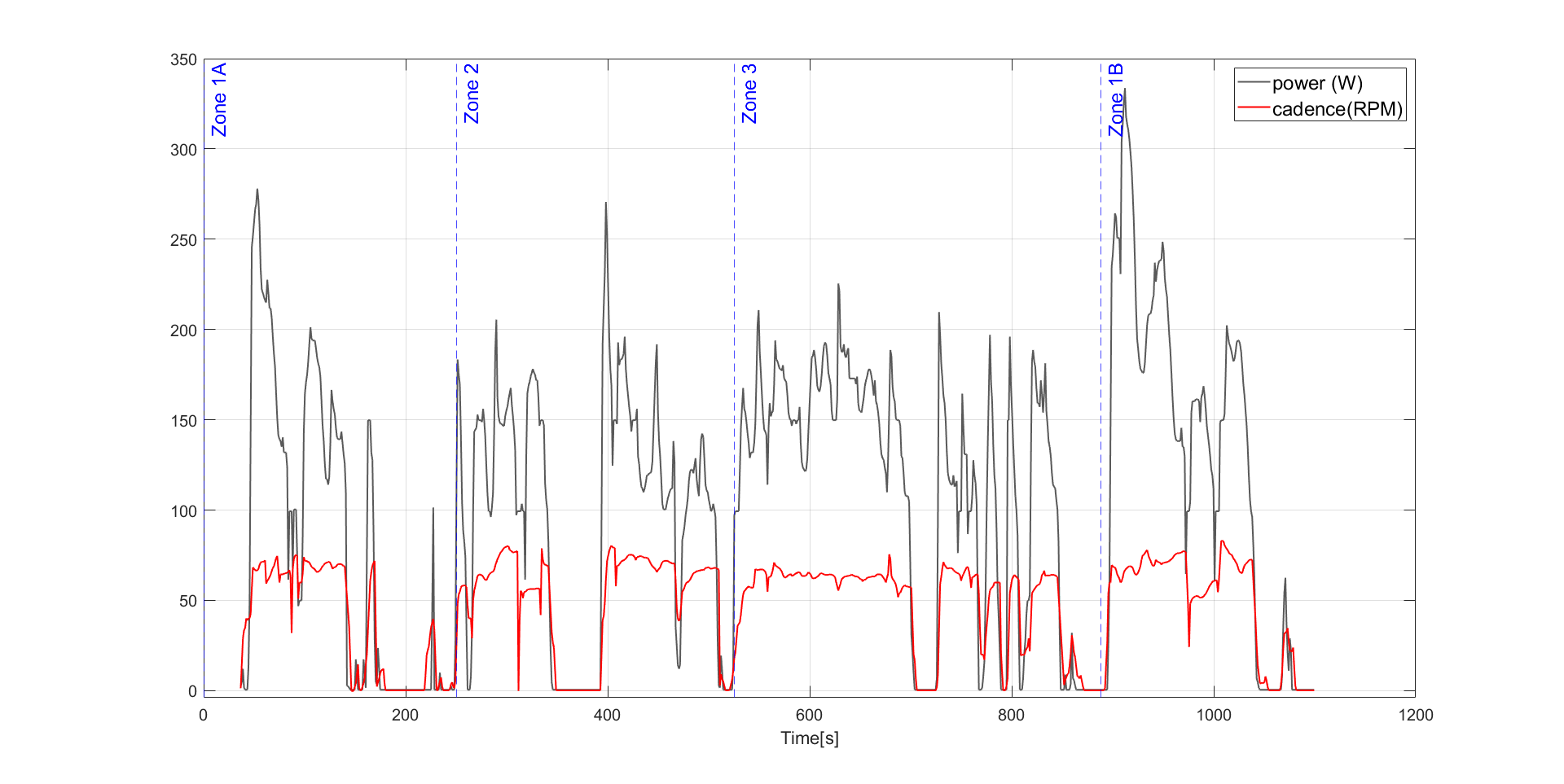
Keywords
Copyright © 2023
Shoman et al. This article is distributed under the terms of the Creative Commons Attribution License (CC BY 4.0), which permits unrestricted use and distribution provided that the original work is properly cited.
Funding
This work is funded by Marie Skłodowska-Curie actions (H2020 MGA MSCA-ITN) within the SAFERUP project under grant agreement number 765057.
Cite this Article
Shoman, M., Imine, H., Johansson, K., & Wallqvist, V. (2023). Measuring Cyclist’s Inputs, the Kinematic and Dynamic Properties of a City Bicycle, and Estimating the Road Profile via Sensor Fusion. Highlights of Vehicles, 1(1), 1–16. https://doi.org/10.54175/hveh1010001
References
1.
Götschi, T., Garrard, J., & Giles-Corti, B. (2016). Cycling as a part of daily life: A review of health perspectives. Transport Reviews, 36(1), 45–71. https://doi.org/10.1080/01441647.2015.1057877
2.
Raser, E., Gaupp-Berghausen, M., Dons, E., Anaya-Boig, E., Avila-Palencia, I., Brand, C., et al. (2018). European cyclists’ travel behavior: differences and similarities between seven European (PASTA) cities. Journal of Transport & Health, 9, 244–252. https://doi.org/10.1016/j.jth.2018.02.006
3.
Pucher, J., Buehler, R., Merom, D., & Bauman, A. (2011). Walking and cycling in the United States, 2001–2009: Evidence from the national household travel surveys. American Journal of Public Health, 101(no. S1), S310–S317. https://doi.org/10.2105/AJPH.2010.300067
4.
World Health Organization. (2018). Global status report on road safety 2018. World Health Organization.
5.
Bucchi, A., Sangiorgi, C., & Vignali, V. (2012). Traffic psychology and driver behavior. Procedia - Social and Behavioral Sciences, 53, 972–979. https://doi.org/10.1016/j.sbspro.2012.09.946
6.
Shoman, M., Simone, A., & Vignali, V. (2018). Looking behavior to vertical road signs on rural roads. MOJ Civil Engineering, 4(2), 75–79. https://doi.org/10.15406/mojce.2018.04.00100
7.
Hoffman, M. R., Lambert, W. E., Peck, E. G., & Mayberry, J. C. (2010). Bicycle commuter injury prevention: it is time to focus on the environment. The Journal of Trauma: Injury, Infection, and Critical Care, 69(5), 1112–1119. https://doi.org/10.1097/TA.0b013e3181f990a1
8.
Observatoire national Interministériel de la sécurité routière. (2021). La sécurité routière en France Bilan de l’accidentalité de l’année 2021 (in Franch). Observatoire national Interministériel de la sécurité routière.
9.
Gadsby A., &. Watkins, K. (2020). Instrumented bikes and their use in studies on transportation behaviour, safety, and maintenance. Transport Reviews, 40(6), 774–795. https://doi.org/10.1080/01441647.2020.1769227
10.
Miah, S., Milonidis, E., Kaparias, I., & Karcanias, N. (2019). An innovative multi-sensor fusion algorithm to enhance positioning accuracy of an instrumented bicycle. IEEE Transactions on Intelligent Transportation Systems, 21(3), 1145–1153. https://doi.org/10.1109/TITS.2019.2902797
11.
Escalona, J. L., & Recuero, A. M. (2012). A bicycle model for education in multibody dynamics and real-time interactive simulation. Multibody System Dynamics, 27, 383–402. https://doi.org/10.1007/s11044-011-9282-7
12.
Escalona, J. L., Kłodowski, A., & Munoz, S. (2018). Validation of multibody modeling and simulation using an instrumented bicycle: from the computer to the road. Multibody System Dynamics, 43, 297–319. https://doi.org/10.1007/s11044-018-9626-7
13.
Etemad, H., Costello, S. B., Wilson, D. J., & Padiyara, S. (2016). Using an instrumented bicycle to help understand cyclists’ perception of risk. Road & Transport Research, 25(3), 75–78.
14.
Qian, X., Moore, J. K., & Niemeier, D. (2020). Predicting bicycle pavement ride quality: Sensor-based statistical model. Journal of Infrastructure Systems, 26(3), 04020033. https://doi.org/10.1061/(ASCE)IS.1943-555X.0000571
15.
Cain, S. M., & Perkins, N. C. (2012). Comparison of experimental data to a model for bicycle steady-state turning. Vehicle System Dynamics, 50(8), 1341–1364. https://doi.org/10.1080/00423114.2011.650181
16.
Dialynas, G., Christoforidis, C., Happee, R., & Schwab, A. (2023). Rider control identification in cycling taking into account steering torque feedback and sensory delays. Vehicle System Dynamics, 61(1), 200–224. https://doi.org/10.1080/00423114.2022.2048865
17.
Murgano, E., Caponetto, R., Pappalardo, G., Cafiso, S. D., & Severino, A. (2021). A novel acceleration signal processing procedure for cycling safety assessment. Sensors, 21(12), 4183. https://doi.org/10.3390/s21124183
18.
Cafiso, S., Pappalardo, G., & Stamatiadis, N. (2021). Observed risk and user perception of road infrastructure safety assessment for cycling mobility. Infrastructures, 6(11), 154. https://doi.org/10.3390/infrastructures6110154
19.
Dozza, M., Werneke, J., & Fernandez, A. (7–8 November 2012). Piloting the naturalistic methodology on bicycles. International Cycling Safety Conference, Helmond, The Netherlands.
20.
Dozza, M., & Werneke, J. (2014). Introducing naturalistic cycling data: What factors influence bicyclists’ safety in the real world? Transportation Research Part F: Traffic Psychology and Behaviour, 24, 83–91. https://doi.org/10.1016/j.trf.2014.04.001
21.
Dozza, M., Piccinini, G. F. B., & Werneke, J. (2016). Using naturalistic data to assess e-cyclist behavior. Transportation Research Part F: Traffic Psychology and Behaviour, 41(Part B), 217–226. https://doi.org/10.1016/j.trf.2015.04.003
22.
Walker, I. (2007). Drivers overtaking bicyclists: Objective data on the effects of riding position, helmet use, vehicle type and apparent gender. Accident Analysis & Prevention, 39(2), 417–425. https://doi.org/10.1016/j.aap.2006.08.010
23.
Walker, I., Garrard, I., & Jowitt, F. (2014). The influence of a bicycle commuter’s appearance on drivers’ overtaking proximities: An on-road test of bicyclist stereotypes, high-visibility clothing and safety aids in the united kingdom. Accident Analysis & Prevention, 64, 69–77. https://doi.org/10.1016/j.aap.2013.11.007
24.
Chuang, K.-H., Hsu, C.-C., Lai, C.-H., Doong, J.-L., & Jeng, M.-C. (2013). The use of a quasi-naturalistic riding method to investigate bicyclists’ behaviors when motorists pass. Accident Analysis & Prevention, 56, 32–41. https://doi.org/10.1016/j.aap.2013.03.029
25.
Parkin, J., Wardman, M., & Page, M. (2008). Estimation of the determinants of bicycle mode share for the journey to work using census data. Transportation, 35, 93–109. https://doi.org/10.1007/s11116-007-9137-5
26.
Welch, P. (1967). The use of fast fourier transform for the estimation of power spectra: A method based on time averaging over short, modified periodograms. IEEE Transactions on Audio Electroacoustics, 15(2), 70–73. https://doi.org/10.1109/TAU.1967.1161901
27.
Gustafsson, F. (1996). Determining the initial states in forward-backward filtering. IEEE Transactions on Signal Processing, 44(4), 988–992. https://doi.org/10.1109/78.492552
28.
Imine, H. (2003). Observation d’états d’un véhicule pour l’estimation du profil dans les traces de roulement (in Franch) [PhD thesis, Versailles-St Quentin en Yvelines]. ABES. https://www.theses.fr/2003VERS0026
29.
Imine, H., Fridman, L., Shraim, H., & Djemai, M. (2011). Sliding mode based analysis and identification of vehicle dynamics (Vol. 414). Springer Science & Business Media.
30.
Shoman, M., & Imine, H. (27–30 April 2020). Modeling and simulation of bicycle dynamics. TRA 2020, the 8th Transportation Research Arena, Helsinki, Finland.
31.
Shoman, M., & Imine, H. (18–22 October 2020). Subjective validity of bicycle simulators. VEHICULAR 2020: The Ninth International Conference on Advances in Vehicular Systems, Technologies and Applications, Porto, Portugal.
32.
Shoman, M. M., & Imine, H. (2021). Bicycle simulator improvement and validation. IEEE Access, 9, 55063–55076. https://doi.org/10.1109/ACCESS.2021.3071214
Metrics
Loading...
Journal Menu
Journal Contact
Highlights of Vehicles
Editorial Office
Highlights of Science
Avenida Madrid, 189-195, 3-3
08014 Barcelona, Spain
08014 Barcelona, Spain
Jene Zhang
Managing Editor